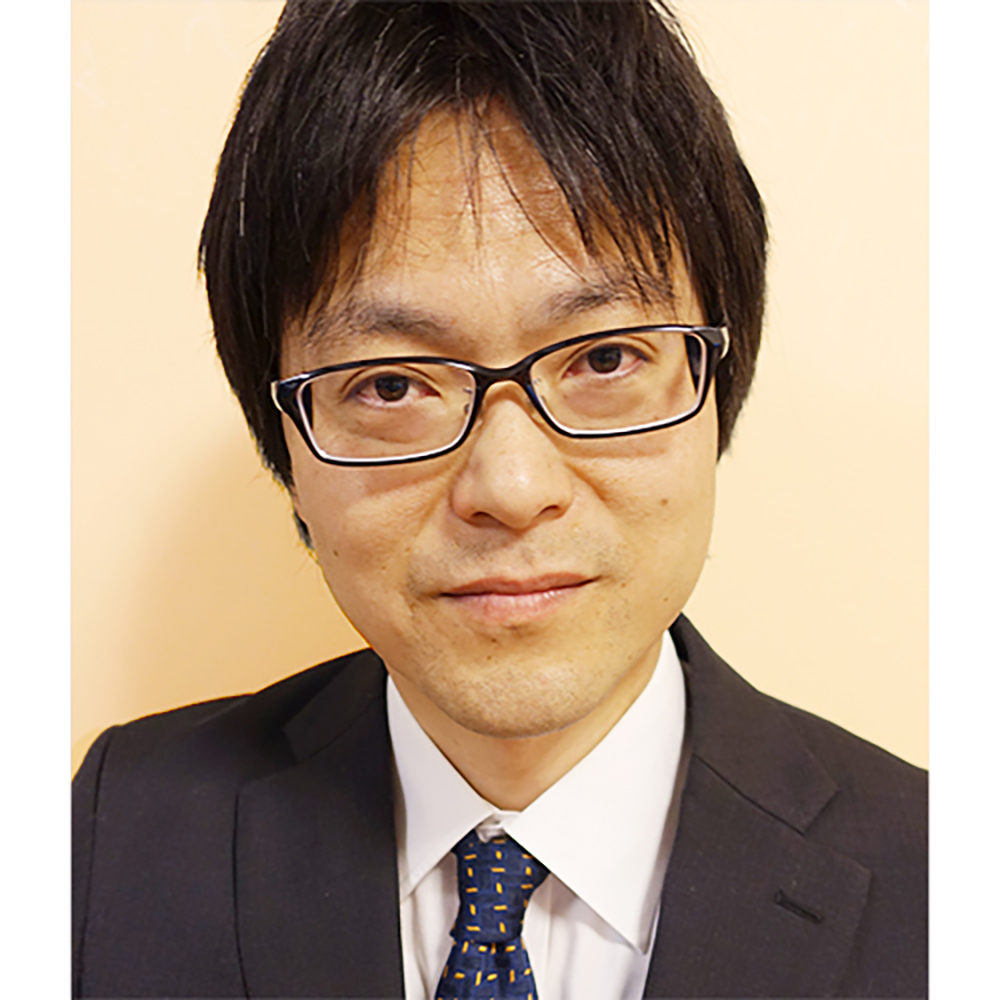
清水 昌平
Position | 滋賀大学データサイエンス学系教授 |
---|---|
Research Field | 統計科学 |
受賞 | 日本行動計量学会 林知己夫賞(優秀賞)(2016) |
着任日 | 2020年4月1日 |
研究概要
因果探索
データから因果関係を推測する方法論である統計的因果推論の研究を行っています。特に,介入を伴う実験によって得られたのではないデータから因果構造を推測する因果探索の研究に取り組んでいます。
科学の主目的は因果関係の解明です. 研究者は, 背景理論に基づく因果仮説を比較・検証するためにデータを用います. また, 背景理論が十分でない場合は, 因果仮説を探索するためにデータを使います. 現在では多種多様で膨大なデータが収集可能になり, また公開されつつあります。しかし, それらのデータは多くの場合, 介入を伴う実験データではありません。
因果関係を調べるためには,介入を伴う実験によって得られるデータが有効ですが、もし介入の伴わない非実験データを用いて, 因果仮説の比較・検証や探索が可能になれば, 背景理論の精緻化や将来の調査・実験デザインに生かすことができ, 因果関係の解明に役立ちます。
本拠点では、遺伝子発現データ等を基に因果関係を推測する方法論の研究およびそれを用いたデータ解析を通じて生命科学の発展に貢献することを目指します。
略歴
2006年に大阪大学基礎工学研究科 博士後期課程修了。2006年-2008年 日本学術振興会特別研究員PD、2008年 東京工業大学においてポスドク研究員、2009年-2016年 大阪大学産業科学研究所において助教・准教授、2016年-2018年 滋賀大学データサイエンス学系において准教授、2018年より滋賀大学データサイエンス学系教授となり現在に至る。
論文
S. Shimizu. Statistical causal discovery: LiNGAM approach. SpringerBriefs in Statistics. Springer Tokyo, 2022.
T. N. Maeda and S. Shimizu. Causal additive models with unobserved variables. In Proceedings of the Thirty-Seventh Conference on Uncertainty in Artificial Intelligence, PMLR 161:97-106, 2021.
T. N. Maeda and S. Shimizu. RCD: Repetitive causal discovery of linear non-Gaussian acyclic models with latent confounders. In Proc. Twenty Third International Conference on Artificial Intelligence and Statistics, PMLR 108:735-745, 2020.
R. Silva and S. Shimizu. Learning instrumental variables with structural and non-Gaussianity assumptions. Journal of Machine Learning Research, 18: 1-49, 2017.
S. Shimizu and K. Bollen. Bayesian estimation of causal direction in acyclic structural equation models with individual-specific confounder variables and non-Gaussian distributions. Journal of Machine Learning Research, 15: 2629-2652, 2014.